Generative AI: Transforming Healthcare with Predictive Diagnostics
Generative AI is making waves in healthcare. One of its most promising applications is in predictive diagnostics. This technology helps doctors spot diseases early and improve patient outcomes.
The Role of Generative AI in Healthcare
Generative AI uses algorithms to analyze large sets of data. It can identify patterns that might be missed by human eyes. This capability is crucial in healthcare, where early detection can save lives.

What Is Generative AI?
Generative AI refers to a class of artificial intelligence systems that can generate new content based on input data. Unlike traditional AI models that focus on classification or detection (e.g., identifying cancer in an X-ray), Generative AI can create new data, simulate scenarios, and even predict outcomes based on patterns learned from historical data.
Generative models, such as Generative Adversarial Networks (GANs), Variational Autoencoders (VAEs), and Transformer-based models, can generate synthetic data, simulate biological processes, or predict disease progression. These capabilities are proving invaluable in healthcare, particularly in predictive diagnostics.
The Role of Predictive Diagnostics in Healthcare
Predictive diagnostics involves using data analytics, machine learning, and other technologies to predict the likelihood of diseases before they fully manifest. Traditionally, diagnostics rely on current symptoms, lab results, and medical history. While this has been effective, there are significant limitations:
- Delayed Detection: Many conditions, such as cancer or Alzheimer’s disease, show symptoms only in later stages, making early detection difficult.
- Inconsistencies: Different healthcare providers may interpret diagnostic data differently, leading to variability in diagnosis.
- One-size-fits-all Approach: Conventional diagnostics often lack personalization, relying on generalized data that may not consider individual genetic or environmental factors.
Predictive diagnostics powered by AI helps overcome these issues by providing earlier, more accurate, and personalized diagnoses.
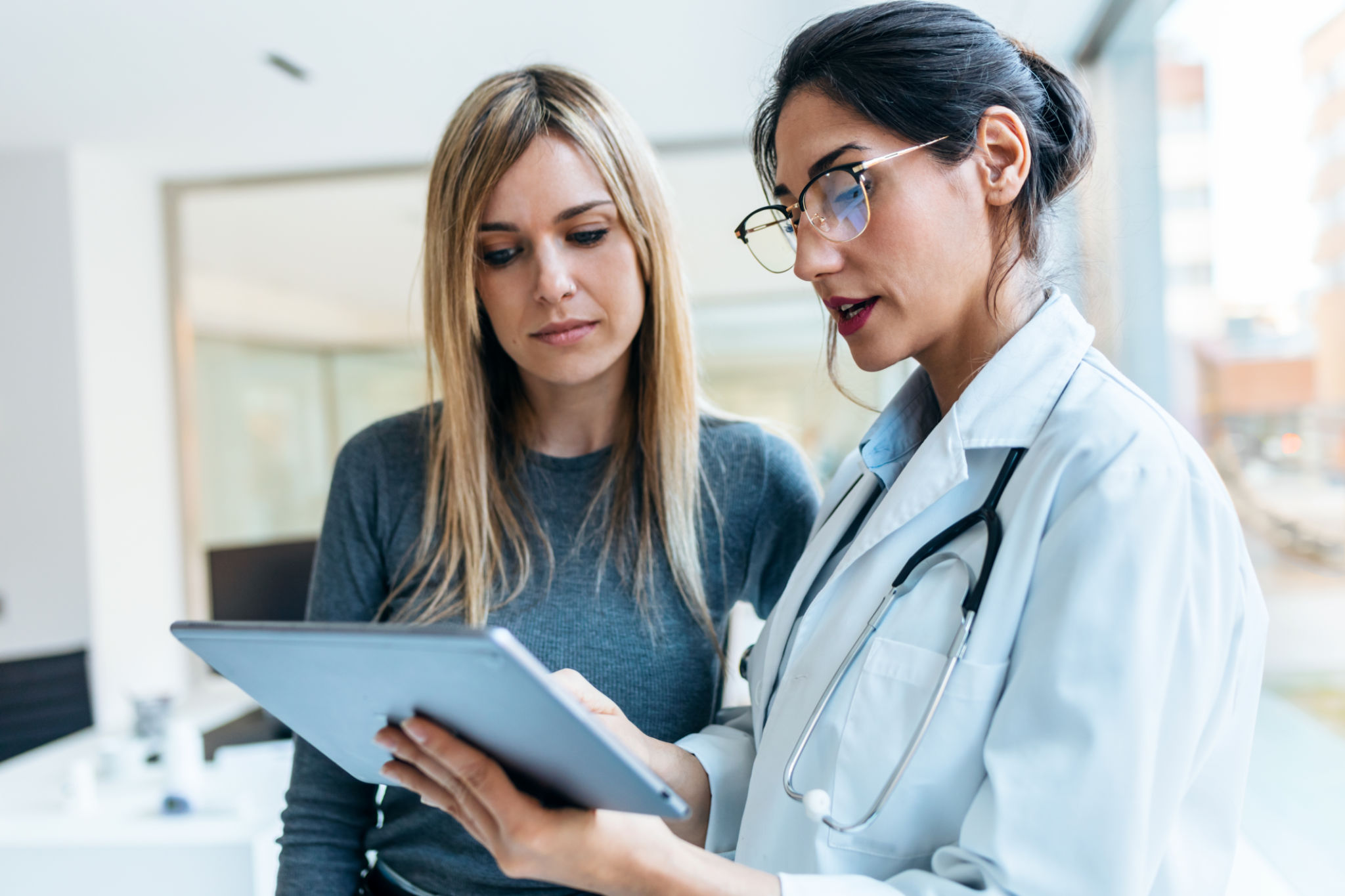
Early Detection of Diseases
Generative AI can identify patterns in large datasets that may not be obvious to human experts. By analyzing genetic data, patient histories, imaging, and biomarkers, Generative AI can predict the likelihood of diseases long before traditional methods would identify them.
For example, AI models trained on genomic data can simulate potential mutations in genes and predict the risk of inherited diseases such as Huntington’s disease or cystic fibrosis. Similarly, for conditions like cancer, Generative AI can detect early-stage changes in imaging that may not yet be detectable by human radiologists.
Personalized Medicine and Predictive Modeling
Every patient’s genetic makeup and lifestyle choices influence how diseases progress and how they respond to treatment. Generative AI helps create personalized disease models by combining medical history, genetic data, and other personal factors. This enables more targeted interventions.
For example, consider a patient at risk of developing heart disease. Generative AI models can simulate how lifestyle changes (such as diet and exercise) might reduce their risk, allowing doctors to tailor recommendations based on highly individualized projections. Similarly, Generative AI can help forecast how a patient might respond to certain medications, reducing the trial-and-error approach common in chronic disease management.
Synthetic Data Generation for Rare Conditions
Rare diseases are often underdiagnosed due to the limited availability of data for training AI models. However, Generative AI can create synthetic datasets that simulate how these rare diseases manifest across different populations. This ability to "generate" new patient cases can help train models that are better equipped to diagnose rare conditions.
For example, researchers working on diagnosing rare genetic disorders can use GANs to create synthetic genetic profiles that mimic real-world patients. This synthetic data expands the available training dataset, enabling AI tools to better recognize and predict these conditions.
Challenges and Ethical Considerations
While Generative AI offers numerous benefits for predictive diagnostics, there are several challenges that must be addressed:
- Data Privacy and Security: The use of patient data, especially genetic information, raises privacy concerns. Ensuring that AI models comply with regulations like HIPAA/AI EU Act is crucial.
- Bias in AI Models: Generative AI models trained on biased datasets may produce inaccurate predictions for certain populations. It is essential to ensure diversity in training data to avoid this issue.
- Clinical Validation: While AI models can provide predictions, these need to be clinically validated to ensure accuracy and effectiveness before being used in real-world medical settings.
While Generative AI offers many benefits, it also comes with challenges. Ensuring data privacy and security is paramount. Healthcare providers must implement robust measures to protect patient information.
Ethical considerations also play a role. AI should complement, not replace, human judgment. Medical professionals must remain involved in the decision-making process to ensure the best outcomes for patients.
Generative AI has the potential to radically improve predictive diagnostics by making healthcare more personalized, proactive, and precise. From early disease detection to personalized medicine and accelerated drug discovery, the applications of Generative AI in healthcare are vast and still expanding. While challenges remain, the benefits of using Generative AI in predictive diagnostics make it one of the most promising developments in modern medicine.
As Generative AI continues to evolve, it’s poised to play a pivotal role in shaping a healthcare system that’s not only reactive but predictive—helping doctors anticipate diseases and save lives before the first symptom even appears.